A BERT Based Method for Continuous Estimation of Cross-Subject Hand Kinematics From Surface Electromyographic Signals
A BERT Based Method for Continuous Estimation of Cross-Subject Hand Kinematics From Surface Electromyographic Signals
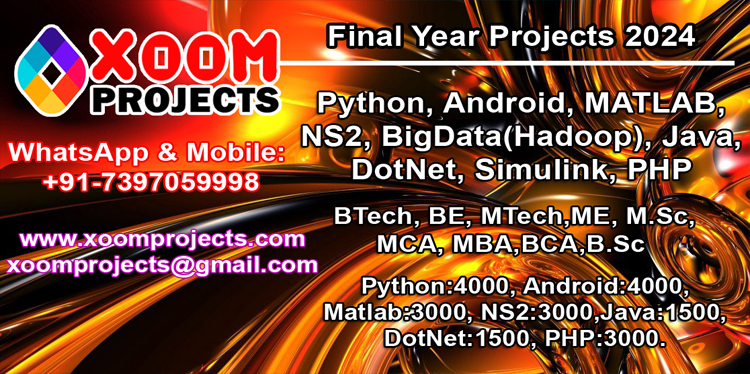
Abstract
Estimation of hand kinematics from surface electromyographic (sEMG)
signals provides a non-invasive human-machine interface. This approach is
usually subject-specific, so that the training on one individual does not
generalise to different subjects. In this paper, we propose a method based on
Bidirectional Encoder Representation from Transformers (BERT) structure to
predict the movement of hands from the root mean square (RMS) feature of
the sEMG signal following μ -law normalization. The method was tested for
within-subject and cross-subject conditions. We trained the model with two
hard sample mining methods, Gradient Harmonizing Mechanism (GHM) and
Online Hard Sample Mining (OHEM). The proposed method was compared
with classic approaches, including long short-term memory (LSTM) and
Temporal Convolutional Network (TCN) as well as a recent method called
Long Exposure Convolutional Memory Network (LE-ConvMN). Correlation
coefficient (CC), normalized root mean square error (NRMSE) and time costs
were used as performance metrics. Our method (sBERT-OHEM) achieved
state-of-the-art performance in cross-subject evaluation as well as high
performance in subject-specific tests on the Ninapro dataset. The above tests
are based on the same randomly selected 10 subjects. Generally, in the
cross-subject situation, with the increasing of the subjects’ number, it
unavoidably leads to the decline of the performance. While the performance of
our method on 38 subjects was significantly higher than the other methods on
10 subjects in cross-subject conditions, which further verified the advantage of
our methods.