A Best Balance Ratio Ordered Feature Selection Methodology for Robust and Fast Statistical Analysis of Memory Designs
A Best Balance Ratio Ordered Feature Selection Methodology for Robust and Fast Statistical Analysis of Memory Designs
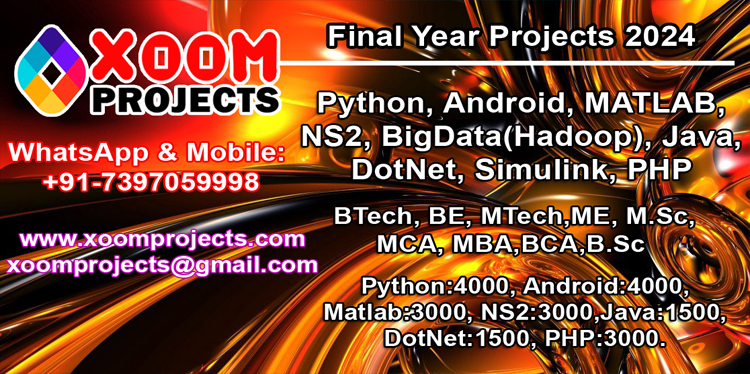
Abstract
Recently, machine learning yield models for integrated circuit (IC) have gained
widespread prominence in the EDA community, and are very promising in
terms of emulating memory design functionality and thereby speeding up
circuit simulation-based variance r
arises in this area is a class imbalance that occurs naturally due to the high
targeted manufacturing yield. Thus, the imbalanced nature of the sampled
memory datasets can compromise the model performance. In this wo
attain deep insights into the memory classification problem for modeling rare
fail events in the context of importance sampling
propose a comprehensive and computationally efficient method that
addresses the joint considerat
reduction methods. A main challenge that
sampling-based yield analysis. We
considerations of the best combination of relevant features
eduction work, we
ions
and class balance ratios, which are key for classifier generalization capability.
The methodology relies on synthetic minority over-sampling techniques to
enforce the minority class while probing for the best data balance ratio in
conjunction with an iterative L1 -SVM-based approach that qualifies as an
approximation to the L0 -norm regularization for the best feature subset
selection. We compare the proposed methodology against standalone L1 -
SVM solutions, unbalanced L0 -norm approximation as well as an algorithmic
data balancing method in the context of yield estimation methodology. The
methodology is shown to result in high fidelity classifiers as demonstrated
when analyzing the yield of a 14-nm FinFET SRAM cross-section with
speedup of 179× for the importance sampling simulations compared to pure
circuit simulation-based approaches and an average error of 0.19σ