A Clinical Decision Support System for Heart Disease Prediction Using Deep Learning
A Clinical Decision Support System for Heart Disease Prediction Using Deep Learning
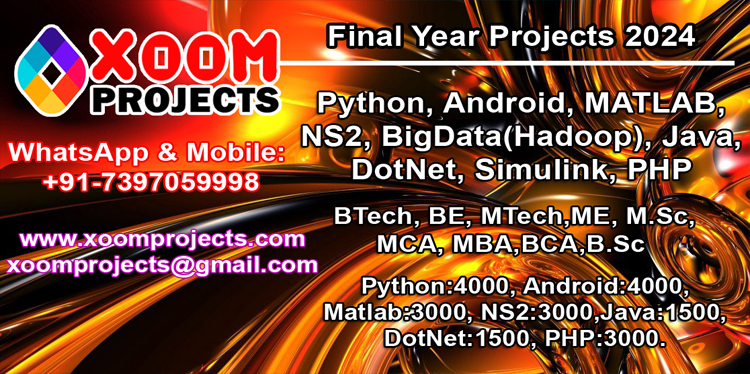
ABSTRACT
Unfortunately, heart disease is currently the primary cause of mortality worldwide and its
incidence is increasing. Detecting heart disease in its initial stages before a cardiac event takes place poses
challenges. Huge amount of heart disease data is available in the health care sector such as in clinics,
hospitals etc. However, this data is not intelligently handled to identify the hidden patterns. Machine
learning techniques help in turning this medical data into useful knowledge. Machine learning is used
to design such decision support systems (DSS) that can learn and improve from their past experiences.
Recently, deep learning has gained the interest of industry and academics. The fundamental objective of this
research activity is the precise diagnosis of heart illness. The suggested approach makes use of a Kerasbased
deep learning model to compute results with a dense neural network. The proposed model undergoes
testing with various configurations of hidden layers in the dense neural network, ranging from 3 layers to
9 layers. Each hidden layer employs 100 neurons and utilizes the Relu activation function. To carry out
the analysis, several heart disease datasets are utilized as benchmarks. The assessment encompasses both
individual and ensemble models, and is performed on all heart disease datasets. Furthermore, using important
measures like sensitivity, specificity, accuracy, and f-measure, the dense neural network is assessed across
all datasets. The performance of different layer combinations varies across datasets due to varying attribute
categories. Through extensive experimentation, the results of the proposed framework are analyzed. The
study’s conclusions show that, when applied to all heart disease datasets, the deep learning model suggested
in this research paper achieves superior accuracy, sensitivity, and specificity compared to individual models
and alternative ensemble approaches.