A Blockchain- Management and Control Framework Driven by Deep Reinforcement Learning
A Blockchain- Management and Control Framework Driven by Deep Reinforcement Learning
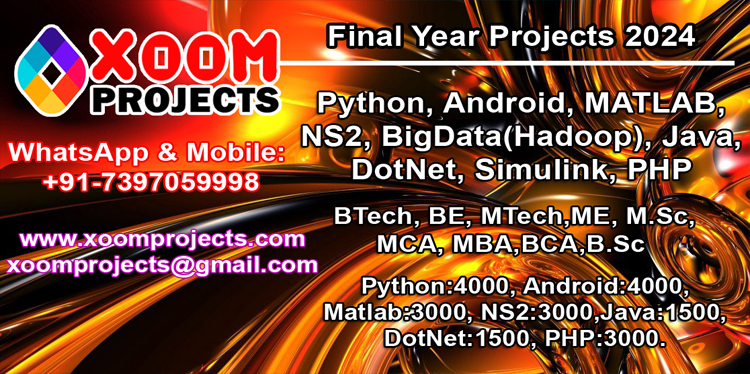
Abstract
The rapid development of Internet
of grid-connected distributed controllable resources (DCR; e.g., electric
vehicles, controllable loads) to provide service to the grid, such as frequency
regulation and demand response. The integration of these DCRs may become
a large virtual power plant network with various characteristics. This poses
great challenges from both control and management perspectives, e.g.,
computation/communication burden, optimization complexity,
limitation, prosumer privacy, etc. In this article, we propose an effective
autonomous incentive-based DCR control and management framework to
integrate a large amount of DCRs to provide grid services, which
simultaneously provides accurate act
optimizes DCR allocations, and maximizes the profits for all prosumers and
system operators. A model
-Enabled Demand
Internet-of-Things in smart grid has enabled millions
esponse. active power adjustment to the grid,
model-free deep deterministic policy gradient
scalability
ive gradient-based
method is designed to find the optimal incentives in a continuous action space
to encourage prosumers to adjust their power consumptions. The method is
implemented in a consortium open-source blockchain platform, Hyperledger
Fabric, which facilitates controls and transaction management. To
demonstrate the effectiveness of the framework, extensive experimental
studies are conducted using real-world data.