A Benchmark Dataset of Endoscopic Images and Novel Deep Learning Method to Detect Intestinal Metaplasia and Gastritis Atrophy
A Benchmark Dataset of Endoscopic Images and Novel Deep Learning Method to Detect Intestinal Metaplasia and Gastritis Atrophy
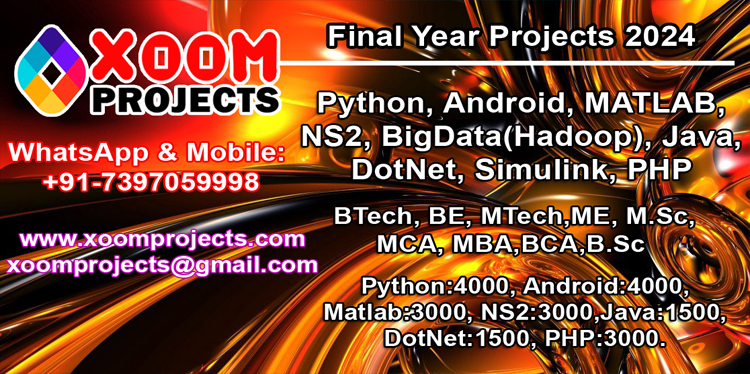
Abstract
Endoscopy has been routinely used to diagnose stomach diseases including
intestinal metaplasia (IM) and gastritis atrophy (GA). Such routine examination
usually demands highly skilled radiologists to focus on a single patient with
substantial time, causing the following two key challenges: 1) the dependency
on the radiologist's experience leading to inconsistent diagnosis results across
different radiologists; 2) limited examination efficiency due to the demanding
time and energy consumption to the radiolog
address these two issues in endoscopy using novel machine learning method
in three-folds. Firstly, we build a novel and relatively big endoscopy dataset of
21,420 images from the widely used White Light Imaging (WLI) endoscopy
and more recent Linked Color Imaging (LCI) endoscopy, which were
radiologist. This paper proposes to
nd ist.
annotated by experienced radiologists and validated with biopsy results,
presenting a benchmark dataset. Secondly, we propose a novel machine
learning model inspired by the human visual system, named as local attention
grouping, to effectively extract key visual features, which is further improved
by learning from multiple randomly selected regional images via ensemble
learning. Such a method avoids the significant problem in the deep learning
methods that decrease the resolution of original images to reduce the size of
input samples, which would remove smaller lesions in endoscopy images.
Finally, we propose a dual transfer learning strategy to train the model with
co-distributed features between WLI and LCI images to further improve the
performance. The experiment results, measured by accuracy, specificity,
sensitivity, positive detection rate and negative detection rate, on IM are
99.18 % , 98.90 % , 99.45 % , 99.45 % , 98.91 % , respectively, and on GA
are 97.12 % , 95.34 % , 98.90 % , 98.86 % , 95.50 % , respectively,
achieving state of the art performance that outperforms current mainstream
deep learning models.